
Machine learning refers to technologies that learn how to complete a particular task, based on large amounts of data. Artificial intelligence refers to systems capable of addressing complex problems in ways similar to human logic and reasoning. It can make use of huge amounts of complex data that would be impossible for humans using traditional techniques and it allows expertise to be captured and codified to support repeatable and explicable machine-led decisions.
The use of such technologies in various nuclear sectors was discussed in the publication Artificial Intelligence for Accelerating Nuclear Applications, Science and Technology, which was based on the IAEA’s meeting in 2021. A key chapter discussed its application in the nuclear power industry. It is considered that there are currently five main opportunities for AI to achieve a positive impact in the nuclear power industry.
They are:
- Automation
- Optimisation
- Analytics
- Prediction and prognostics
- Insight
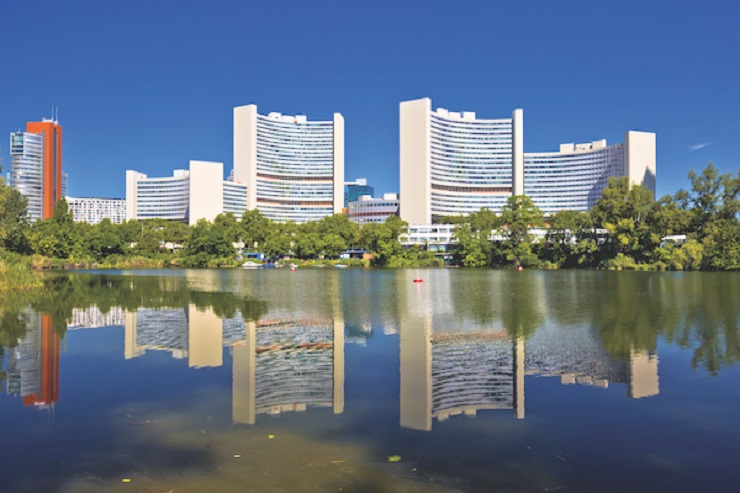
Automation: increasing reliability and speeding up operations
Nuclear plant staff can be placed in demanding situations to complete common tasks, making errors more likely and affecting safety. Other tasks may be repetitive and time-consuming. Automation can help address these issues.
The wide range of established machine learning techniques allows the automation of very different processes, from automated analysis of complex process data to facilitating decision-making and improving work processes. Many of these applications are already well-developed, such as in-service inspection. This is critical to safe operation, but analysing inspection data is laborious, time-consuming and vulnerable to human errors.
Automating data analysis with machine learning improves reliability and efficiency and can extract additional information to improve predictability. For example, control rod drive mechanism (CRDM) coil currents are vulnerable to the build-up of metallic deposits (crud), which can mean the rods are dropped from their grippers, causing downtime. The crud shows up as a current anomaly, but identifying it requires hundreds of person-hours evaluating thousands of measurements. Machine learning can detect anomalies with 96% accuracy in near real-time.
Machine learning can also detect data trends, such as alerting operators to a growing anomaly when it is not visible to the operator and before it results in a significant change. Another application is combining it with drones, to reduce the need for operations staff to walk around the plant to inspect it and collect information.
Human-computer interaction in the control room is the basis for efficient operator perception and control of the operating status of the plant. Here, fast speech and gesture recognition can simplify the process and improve efficiency. Natural language processing can be coupled with machine learning to replicate the human decision-making processes of analysing event reports and generating outcomes. This area of research is rapidly advancing into task-specific tools.
Optimisation: increasing efficiency and managing complex operations
Optimising complex processes such as inventory management, outage scheduling and fuel cycle parameters will improve plant operation. This applies throughout the plant design life cycle, from initial engineering, construction, and operation to decommissioning.
Elements of AI have already been used in building information modelling (BIM) software. What holds up adoption is the lagging regulation for AI application in the plant design engineering process, machine-readable requirements, and data, methodologies and design algorithms that are accessible for AI.
In operation, reactor controllers already take care of some factors such as permitting operation only within given limits, shaping the power density distribution or operation economy. Machine learning can improve core-control methods and improve predictive control. Unlike human controllers, AI can address many different goals simultaneously so for example reactor governance, safety, ergonomics, operation economy and grid services can be processed simultaneously, in accordance with each other.
Analytics: improving models and systems understanding
AI techniques can support research. They can, for instance, speed up the characterisation and validation of materials for new designs, reducing the time and cost of the necessary materials research, as well as helping develop new quality assurance practices for additively manufactured components for small and microreactors. They can optimise the design of experiments to reduce uncertainties, develop advanced dispatch and control methods for nuclear reactors for energy and process heat applications and optimise strategies for hybrid nuclear energy systems.
Existing analytical models are often simplistic, in order to be mathematically tractable, and have too little detail or accuracy to inform decision-making. In contrast AI approaches can be leveraged to develop complex models and provide more accurate predictions.
AI models can also be used for complex and time-consuming statistical modelling such as for fitness-for-service assessments. In this case, traditional models retain an advantage in their higher generalisation to new data given the explicitly defined relations between features and targets. This explicit, direct relationship is typically lacking in AI models, impacting their performance on new data. This shortcoming can be overcome by incorporating physical concepts into the AI model, showing that expert knowledge can be used in conjunction with AI techniques to improve current modelling capabilities.
Finally, AI can be used for model validation, especially for advanced computer simulations that have proliferated over the last years and generate large amounts of data. Such approaches can support, for instance, digital twin applications.
Prediction and prognostics: informing maintenance
Predicting events such as failures and assessing current asset conditions are tools that allow plant owners to plan their maintenance and outage strategies. AI allows operation data streams to be used to schedule maintenance or inspection or for highlighting abnormal conditions.
Advanced simulation tools have become available this century, but operators have to be convinced that they will provide more accurate predictions than existing tools calibrated over decades. Experts are needed to assess whether and how the advanced tools could beat legacy tools but AI can provide mathematically rigorous and explainable algorithms to measure information content available in the simulation and experimental data using information theory principles
Insights from experiments and operation
The nuclear industry has thousands of reactor years of operating experience and huge libraries of support for model validation. Data science technologies can leverage this rich experience in unprecedented ways to unlock new best practices and better inform future decisions, from conceptual design to licensing and operation.
Applications include holistic assessment of maintenance records to extract lessons or identify the best sensor and arrangement for a class of reactors (correlated with various sources of process anomalies, undetected with existing equipment-specific sensors).
In general, methods are in development and are currently exploring how to gain insight from nuclear data. A key challenge is that the methods are both language and jargon-specific. They need an industry dictionary.
Deployment challenges
It is currently challenging to provide interpretability, confidence, and robustness measures of performance for AI and to allay cybersecurity and regulatory concerns.
Cyber attacks used against AI systems aim to have the model make poor decisions and this allows for a large attack surface distributed over time. It is important to proactively address these cyber fears.
Regulators may find traditional assurance inapplicable and the limited transparency of AI and machine learning may make actions difficult to interpret, biases unclear and malfunctions mysterious. Moreover, it is much more fast-moving than regulatory processes. High-level regulatory safety assessment principles and guidance are required and so are standards.
This article first appeared in Nuclear Engineering International magazine.